Hello, I’m Ylli Bajraktari, CEO of the Special Competitive Studies Project. In this week’s edition of 2-2-2, SCSP’s Pieter Garicano, Nina Badger, and Nicholas Furst discuss AI power demand forecasts. We will be hosting the AI + Energy Summit on this topic on September 26, 2024. We are also excited to announce the participation of Robert M. Blue (CEO, Dominion Energy), Charles Meyers (Executive Chairman, Equinix), and Ali Zaidi (National Climate Advisor, White House Climate Policy Office) as our first set of speakers.
Click here to sign up to attend the summit. After Energy, we will convene the AI + Robotics Summit on October 23, 2024. Stay tuned to see who else will join us!
How Bad Will the AI Power Crunch Be?
To win the AI race, America needs to be able to supply its data centers with a sufficient amount of electricity. Before it can do that, it has to know how much is needed.
Recent estimates from the Federal Energy Regulatory Commission (FERC) predict sizable but not overwhelming load increases. Its staff expects data center usage in the United States to grow from 17 gigawatts (GW) in 2022 to 35 GW in 2030 (a GW is roughly equivalent to the power used by 750,000 American homes). The FERC’s projection works out to an average annual growth rate of 9%. Goldman Sachs has projected that AI power use — a narrower category than data centers overall — will grow more rapidly still, from 4 terawatt-hours (TWh) in 2023 to 93 TWh in 2030: an average growth rate of roughly 57% per year.
These assessments are significant: 93 TWh is more than all the power used by the State of Washington in 2022 — equivalent to a bit over 10 GW of installed capacity. But the forecasts pale in comparison to those of actors closer to the AI industry. SemiAnalysis, a chip research group, estimates that AI accelerators will demand more than 10 GW of installed capacity by early 2025, not 2030. That capacity is equal to the power demanded by 7.3 million H100 chips — of which Nvidia will have shipped over 5 million by late 2024.
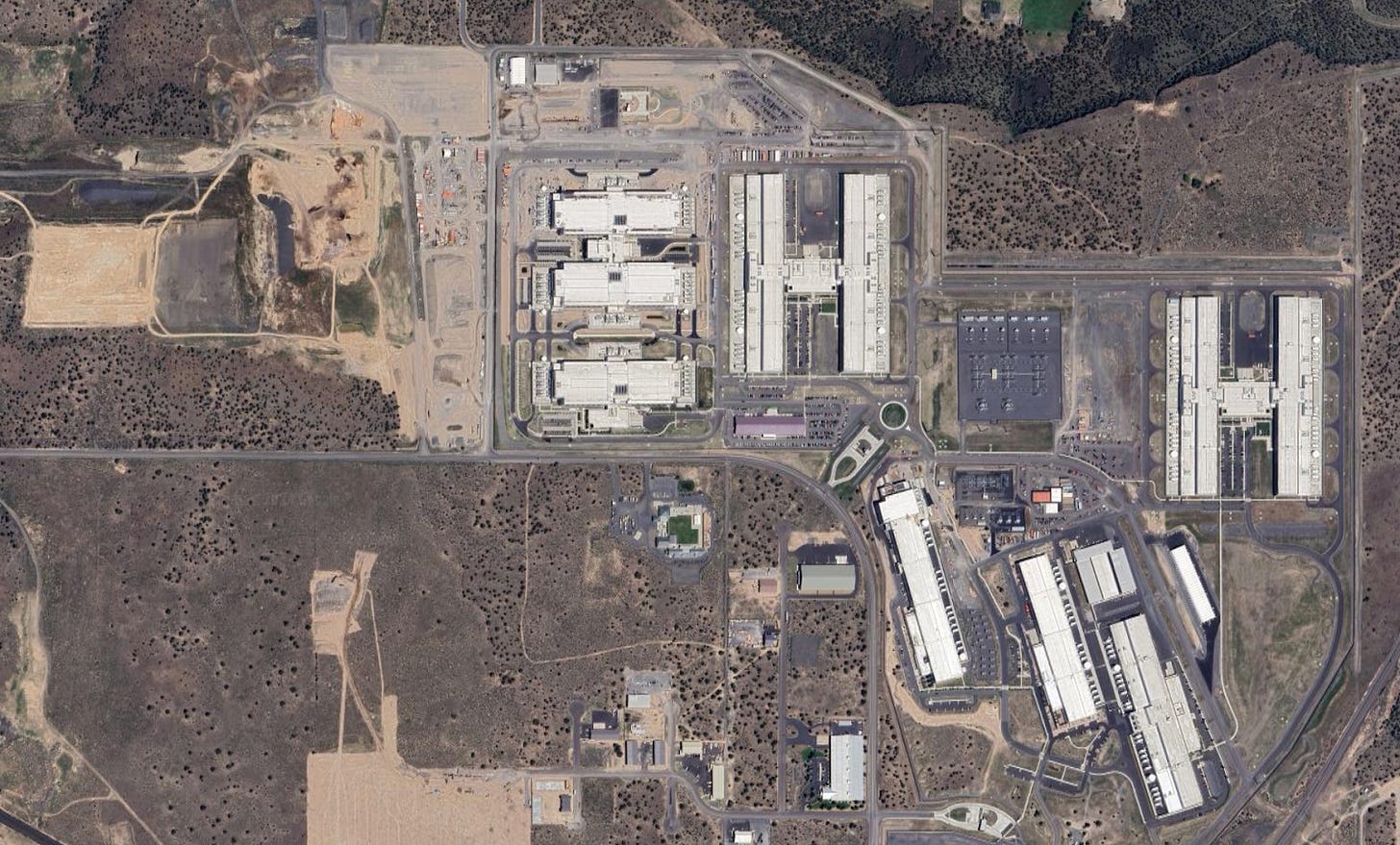
By using property records, power consumption data, and satellite imagery analysis to track major U.S. data center developments, SemiAnalyis concludes that the amount of electricity dedicated to compute for AI alone in U.S. data centers will grow from 3 GW in 2023 to over 56 GW (roughly 400 TWh) by 2028, with total data centers 83 GW — vastly outpacing the FERC’s 35 GW in 2030 projection.
A fourth estimation relies on the growth rate of AI models themselves. According to Epoch AI, an industry tracker, the amount of raw compute being used by leading-edge models is growing by 310% each year. Even if chips continue to become 25% more energy efficient per year, this gives a roughly 228% annualized growth rate in energy consumption of the largest models. A different estimate in May projected leading-edge AI training compute to grow by 0.5 orders of magnitude per year — equivalent to a 216% annual growth rate. On this trend, leading data centers could use 10 GW by 2028 — equivalent to the power use of Connecticut — and 100 GW by 2030.
Extrapolating from the current training growth rate has significant downsides. It assumes that demand for cutting-edge capabilities will remain as high as it is for existing models. It does not account for how many labs would seek to provide large-scale, state-of-the-art models to customers. However, given that state-of-the-art training runs are currently centralized, it gives us a strong sense of how severe the local power crunch will become — and how steep the take-off could feasibly be.
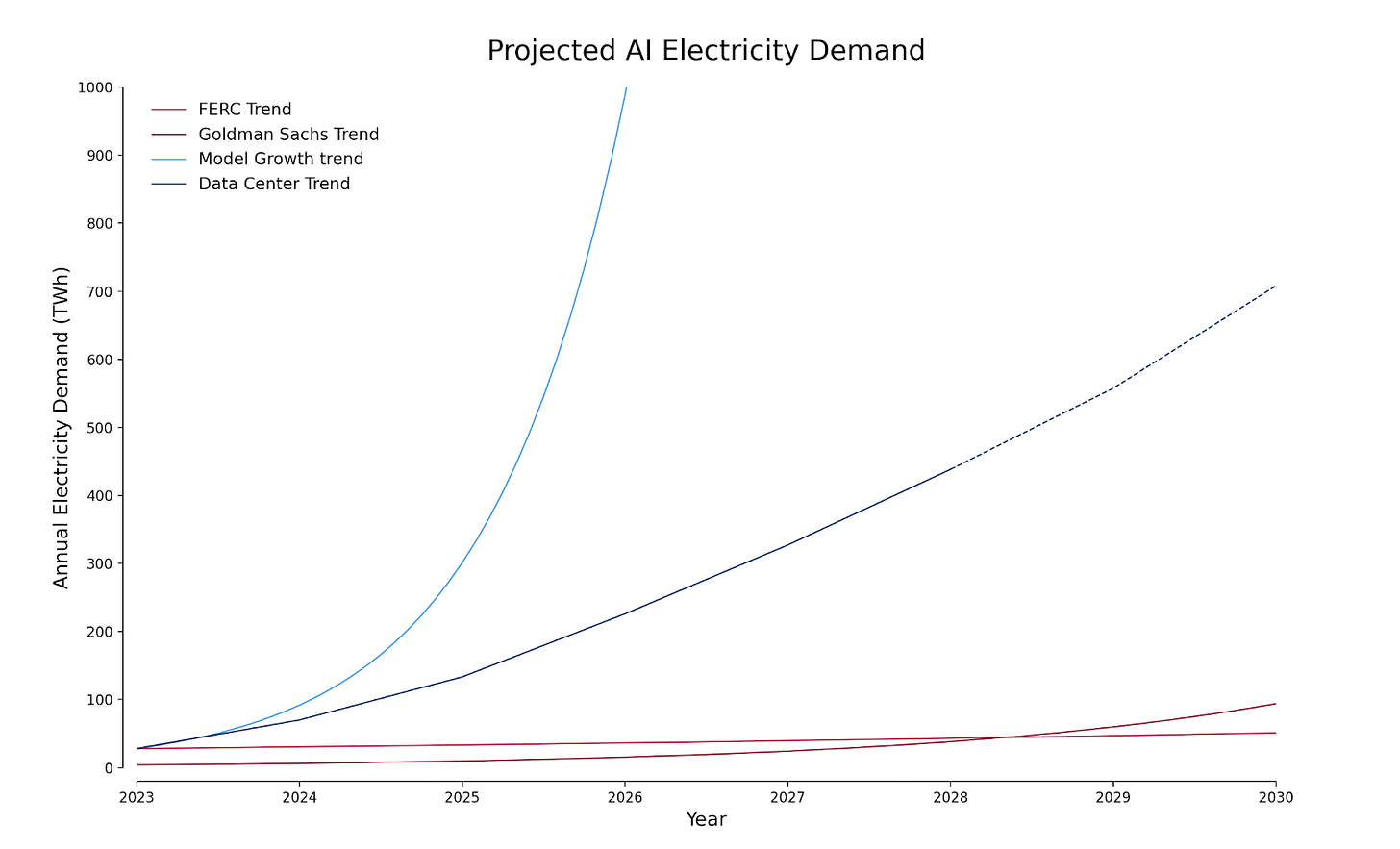
Inference
A number of changes will disrupt these trends. Much of the coverage so far has focused on the power required to train models. But as capabilities improve and AI adoption grows, inference — the computations taking place when a user queries an AI model — is set to grow rapidly as well. Current estimates place the optimal mix of compute allocated to training and to inference at 1:1. But as inference demand rises, the true long-term cost of diffused AI could be multiples higher than current projections.1
To try and construct an estimate of future inference costs, one can focus on the demand side. One of the more well-defined use cases for AI is in software engineering. Setting aside AI’s other existing applications (e.g., customer service and legal writing) and potential future capabilities (e.g., advanced creative writing), modeling the power consumption scenario where every software engineer in the United States uses five AI agents in their workflow sets a plausible lower bound for inference costs after widespread AI adoption.
Previous research using LLAMA 65B estimated that a token of text generation consumes up to 3-4 joules, or about 0.001 Wh. If we apply this to roughly 1.7 million U.S. software engineers using multiple agents that each run hundreds of queries per day, the energy cost of inference could quickly approach hundreds of terawatt-hours per year.2
If anything, this estimate likely undershoots the true expansion of inference demand. The Jevons paradox, first posited during the Industrial Revolution, holds that efficiency gains increase the use of a resource, potentially offsetting the expected savings. As we find more uses for LLMs, and can employ them more quickly and easily (as might happen if everyone has coding agents), there will likely be an enormous proliferation of software.3 Training growth is predictable, the increase in inference driven by changing user habits is not.
Energy Innovation
A second disruption to current trends will be energy efficiency gains. As the relative price of energy rises, energy-saving innovations will be at a premium. In 1999, a popular article estimated that the Internet would use over half of all power before the end of the next decade. In reality, it consumed a sliver of that, around 2%, in large part driven by enormous increases in chip efficiency.
There are reasons to think that this time may be different. Many researchers believe Moore’s Law, the prediction that the number of transistors on a chip (and thus, compute power) would double every 18 to 24 months, is coming to an end as leading-edge chips reach atomic scales. For decades, energy usage associated with compute scaled down alongside Moore’s Law (a phenomenon known as Dennard Scaling). The collapse of this trend in the mid-2000s has led to today’s dramatic increase in energy consumption. New computational paradigms could offer a potential wildcard to meet AI-driven energy demand, but developing and scaling them would require a moonshot effort.
Lastly, many recent efficiency gains have been driven by decreasing the share of electricity that data centers use for non-IT equipment, a ratio known as power usage effectiveness, or PUE. But as PUE continues to decrease — with many hyperscalers below 1.5, and Google claiming to have reached 1.1 PUE — gains will slow down, and eventually hit a wall: a PUE lower than 1 is impossible.4
Policy
In short, AI power demand is growing massively — but with large error bars. For the energy industry, uncertainty is a challenge in its own right. Utilities rely on multi-year development cycles. Investments are made over a period of years, and depreciated over decades.5 The average time it takes to site, permit, and construct an overhead transmission line is 10 years. Given the sizes of the investments involved (even a 1 GW gas plant can cost over $1 billion to build), and the risk aversion usually associated with the energy business, it would be unsurprising if many utilities would under- rather than overshoot data center energy growth projections.
Multiple policy fixes need to be considered. The siting, permitting, and licensing processes for power plants and transmission are extraordinarily lengthy and complex. Streamlining them is an urgent priority. Among the most impactful reforms, a revision of the NEPA process, which forces construction through time-consuming and costly reviews, stands out as a critical step (see recommendation 5.1 in SCSP’s Next-Generation Energy Action Plan).
The scale and type of AI power will create transmission and distribution challenges. For smaller clusters that rely heavily on existing distribution networks, grid modernization efforts, including the deployment of smart grid technologies, will be crucial in improving efficiency and responsiveness across the board. As seen with the recent Amazon-Talen deal, where AWS bought a 960-megawatt data center campus co-located with a nuclear power plant, hyperscalers are increasingly negotiating behind-the-meter deals, essentially bypassing the traditional grid.
As the buildout accelerates, local pushback against data center construction could be a growing factor that could significantly impact AI power availability. Legal challenges contribute to permitting-related delays. Loudoun County, home to the largest concentration of data centers on the globe, is considering a bill to remove by-right use for data centers, requiring county approval for all new developments. Reaching national AI goals will require aligning incentives across state, local and national politics.
The last ingredient may be the ability to manage the uncertainty around estimates. The initial AI power surge caught utilities and investors by surprise. Enabling the rapid construction and interconnection of power plants — giving power generators a chance to respond to short-term changes — should be a national priority. But just as much work should be done to understand the requisite scale of power buildout.
Policymakers need better data to understand the need for reform. Much of the information surrounding power usage and model adoption is currently proprietary. Developing sophisticated forecasting models, and tracking AI energy consumption will be key — a role well-suited to the National Labs and NIST. Fostering closer collaboration between utilities, AI companies, and policymakers will be of similar importance. For this purpose, SCSP will be hosting an upcoming AI + Energy Summit to convene utilities, technologists, hyperscalers, and policymakers in D.C. on September 26.
To understand the surge today, our best approach is looking at the trends in the tech industry — data center construction and model adoption. It is imperative that America creates an energy ecosystem that can respond to tech-driven fluctuations in electricity demand and rapidly build out power. Winning the AI race will mean giving the energy ecosystem the ability to deal with uncertainty, and reducing the uncertainty around AI energy demand.
The reasoning being that marginal improvements to compute performance decrease with scale. For further details, see this paper by Ege Erdil at Epoch AI.
E.g., 1,700,000 software engineers * 5 Agents * 365 days * 1440 minutes per day * 30 queries per minute * 4000 tokens per query * 0.001 Wh ≈ 536 TWh. At least initially, agents may be insufficiently proficient to work 24/7 without human supervision.
E.g., one can think of ‘efficiency gains’ as the increased speed with which a software engineer can write high-quality code by using an LLM, and ‘expected savings’ as the time the engineer otherwise would have spent writing the code manually. If the Jevons paradox holds true, then the proliferation of coding agents may counterintuitively cause engineers to spend more time coding rather than less, as there is more to be coded.
PUE = (power used by a data center as a whole)/(power used for compute). A PUE under 1 would imply that the data center uses less power overall than just one of its components.
This is one of the reasons why climate activists are so worried about utilities locking into gas buildouts in order to meet AI energy demand.
يمكن ان يكون للمشكل الواحد اكثر من حل،ولكن في الواقع العملي الذي لايزال غائبا عن إدراكنا العلمي والتقني لن يكون إلا خيارا واحدا وهو الاسمى ،ان ما تتخبط فيه التكنولوجيا اليوم عايد بالاساس إلى هذا القصور ،قصور نكتشفه عمليا ونتدارك تجاوزه بالبحث العلمي ،للارتقاء و التطوير،وهذا ما يمشي على الامن السيبراني ،وان المشكل تقني وآلي وليس امرا في النفس و العقل البشري فإن التحكم في الآلة اسهل ما يكون ،فلا القوانين و لا الأدبيات تستطيع الخد من المعضلة ،ذلك ان العيب في الآلة والتقنية هو منفذ دخول التصرفات والممارسات الغريبة،فالفم المسدود لا يدخله الذباب،لهذا اصدقائي الاعزاء هناك مشكل تقني في العمل على تامين الحقل السيبراني لا اقل ولا اكثر ولن ياتي الخل من مكان اخر،اذا كنتم مستعدين للتعاون ورفع هذا التحدي من اجل عالم مشترك آمن لا تترددوا في اعتماد تقنيات IMm
مع خالص التحيات
OUCHAMCH MUSTAPHA
The article rightly calls attention to growing AI energy use, and documents widely varying projections. However, a nod to Jevons paradox is insufficient rebuttal to efficiency gains. As the authors note, the internet did *not* consume half of all energy use, but only 2%. Missing here are substantial efforts at efficiency in AI, especially for inference, which is the main worry as use proliferates. Specialized chips like Groq's claim 10x efficiency over GPUs on existing algorithms, and new algorithms that avoid matrix multiplications (Zhu et al 2024) claim inference at ~13 watts on FPGA hardware, close to what the human brain uses.